Risk on default Prediction
With this data analysis I was able to determine the
likelihood of a new customer having future defaults on a loan.
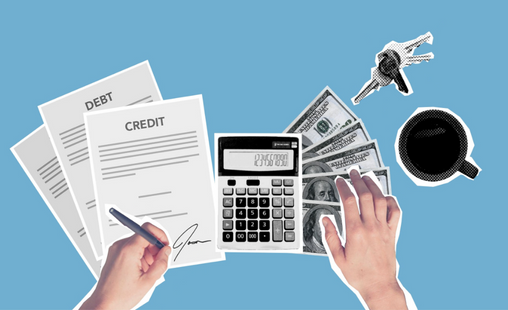
The data for this project were taken from Kaggle and can be downloaded directly from here.
Data Preview
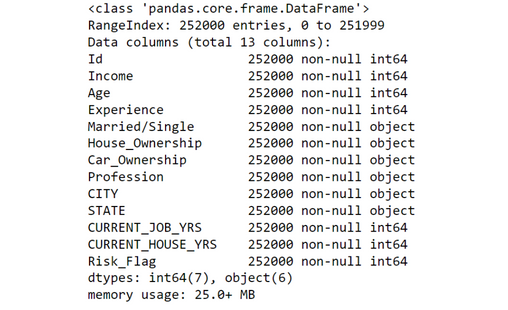



Observations
- The data contain 252000 rows and 13 columns
- There are no NaN values
- There are no duplicate values
Data Dictionary
Variable | Definition |
---|---|
Id | Id of the costumer |
income | Income of the customer |
age | Age of the customer |
experience | Experience of the customer in years |
profession | Profession of the customer |
married | Whether married or single |
house_ownership | House ownership status of the customer |
car_ownership | Whether the customer owns a car or not |
risk_flag | Whether the customer defaulted on the loan or not |
currentjobyears | Years of experience in the current job of customer |
currenthouseyears | Number of years in the current residence |
city | City of residence |
state | State of residence |
Costumer review
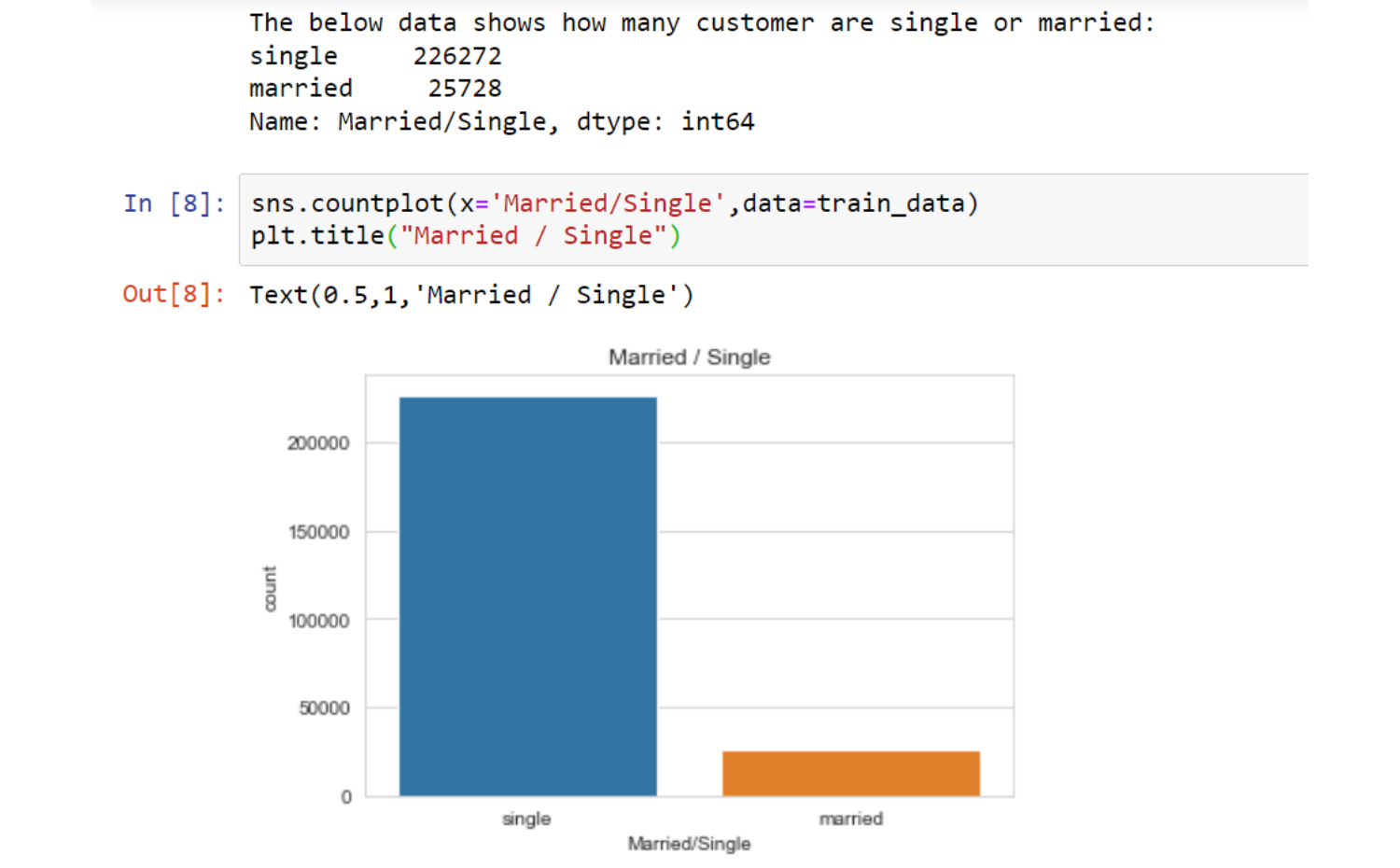
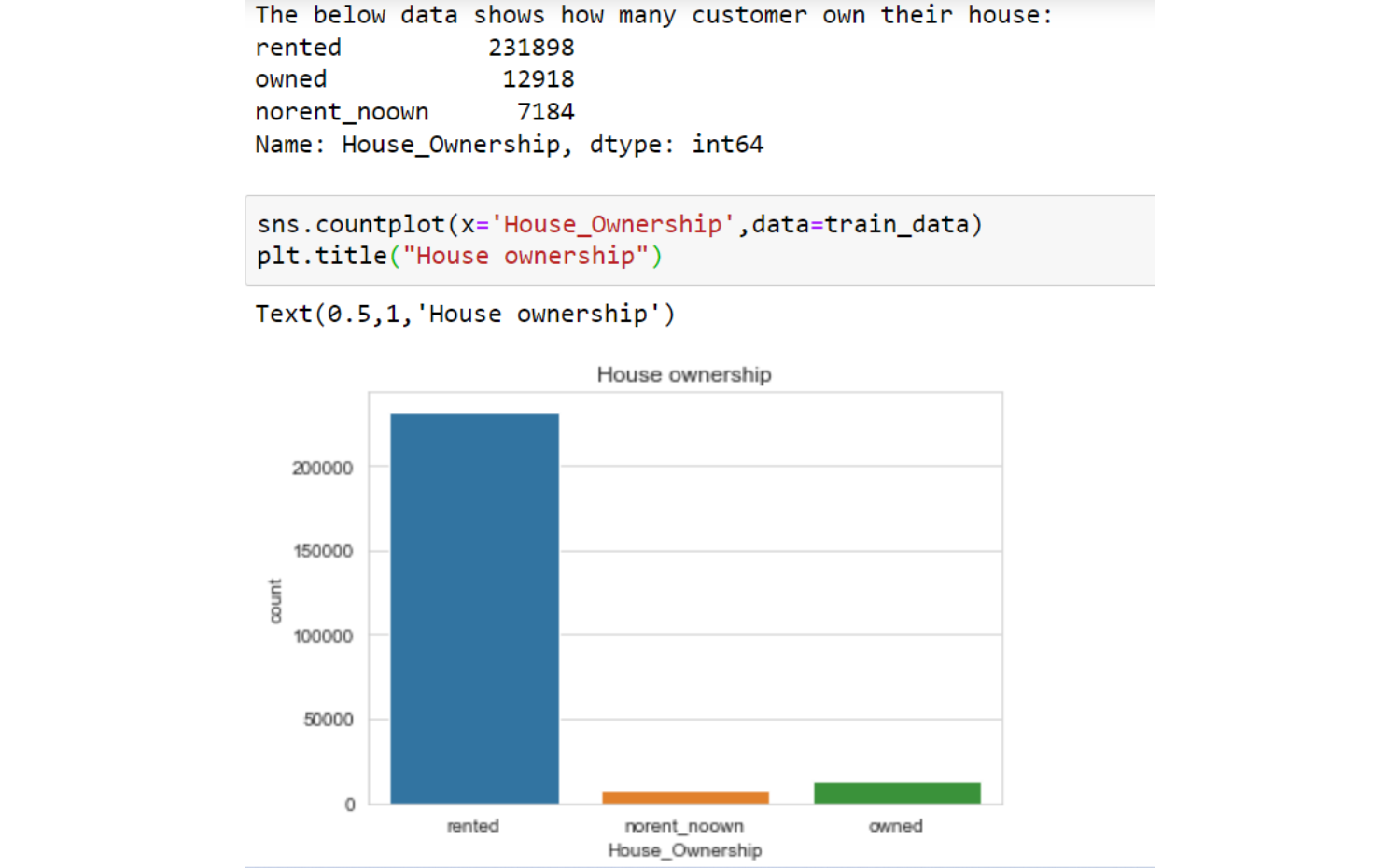
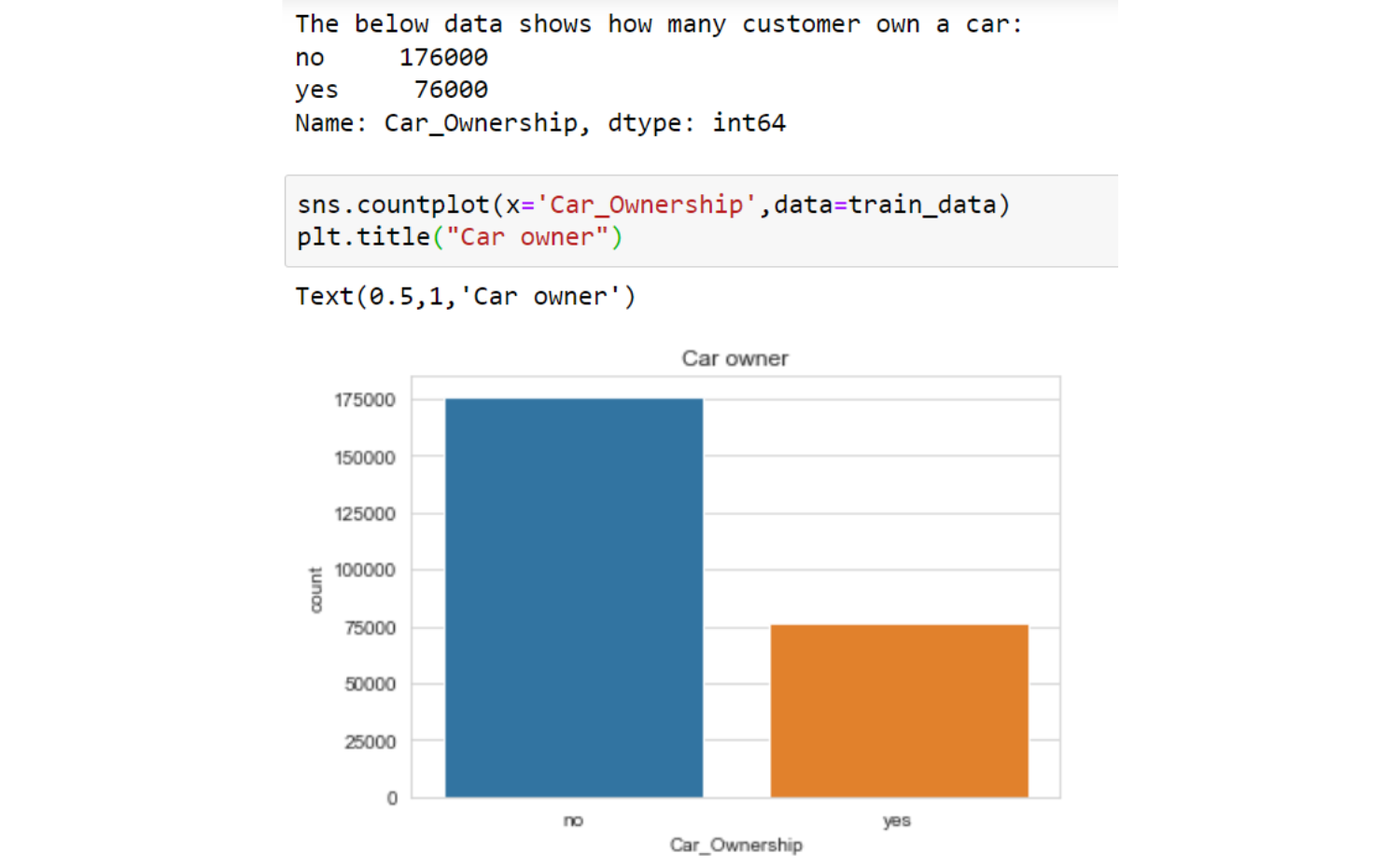
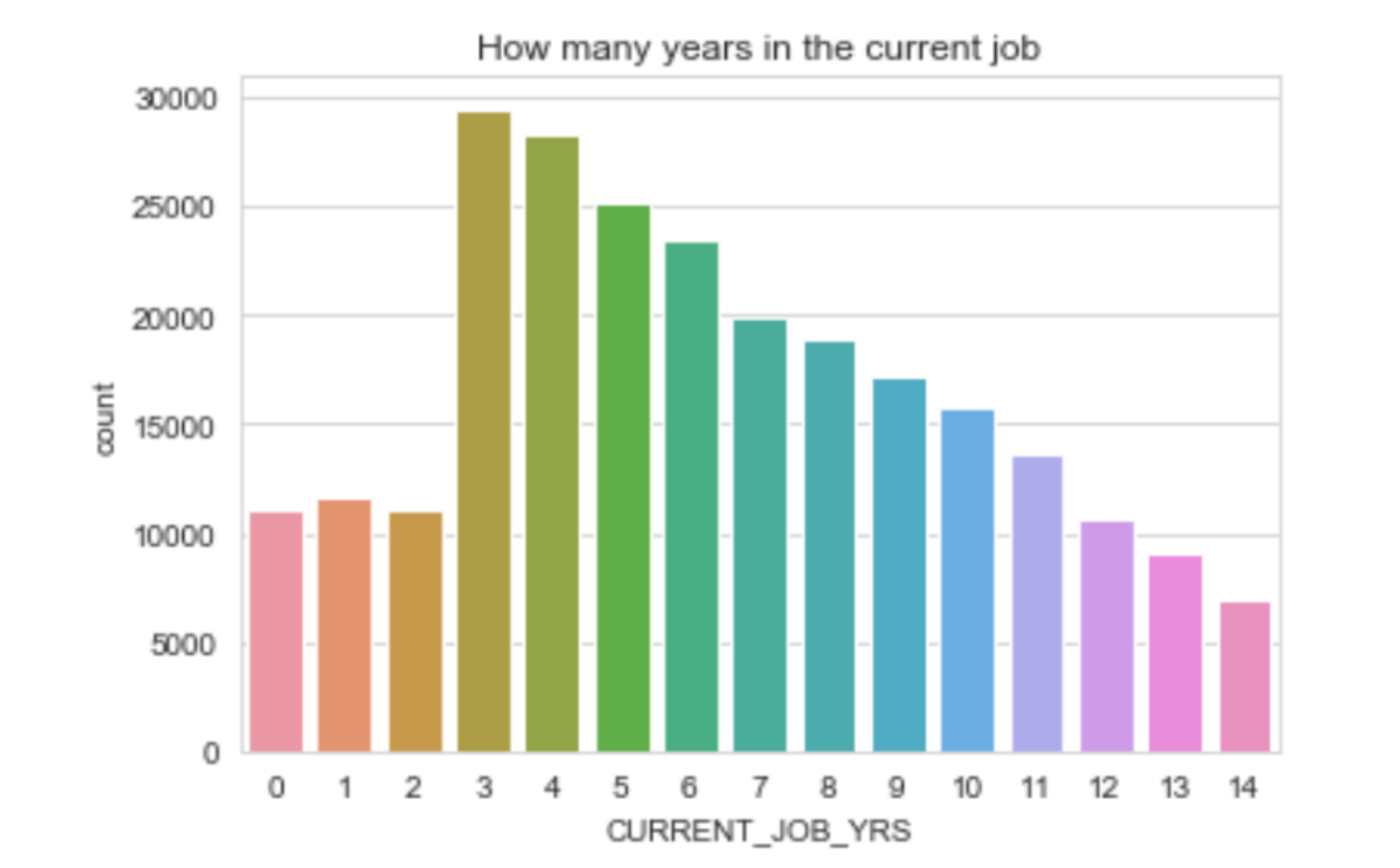
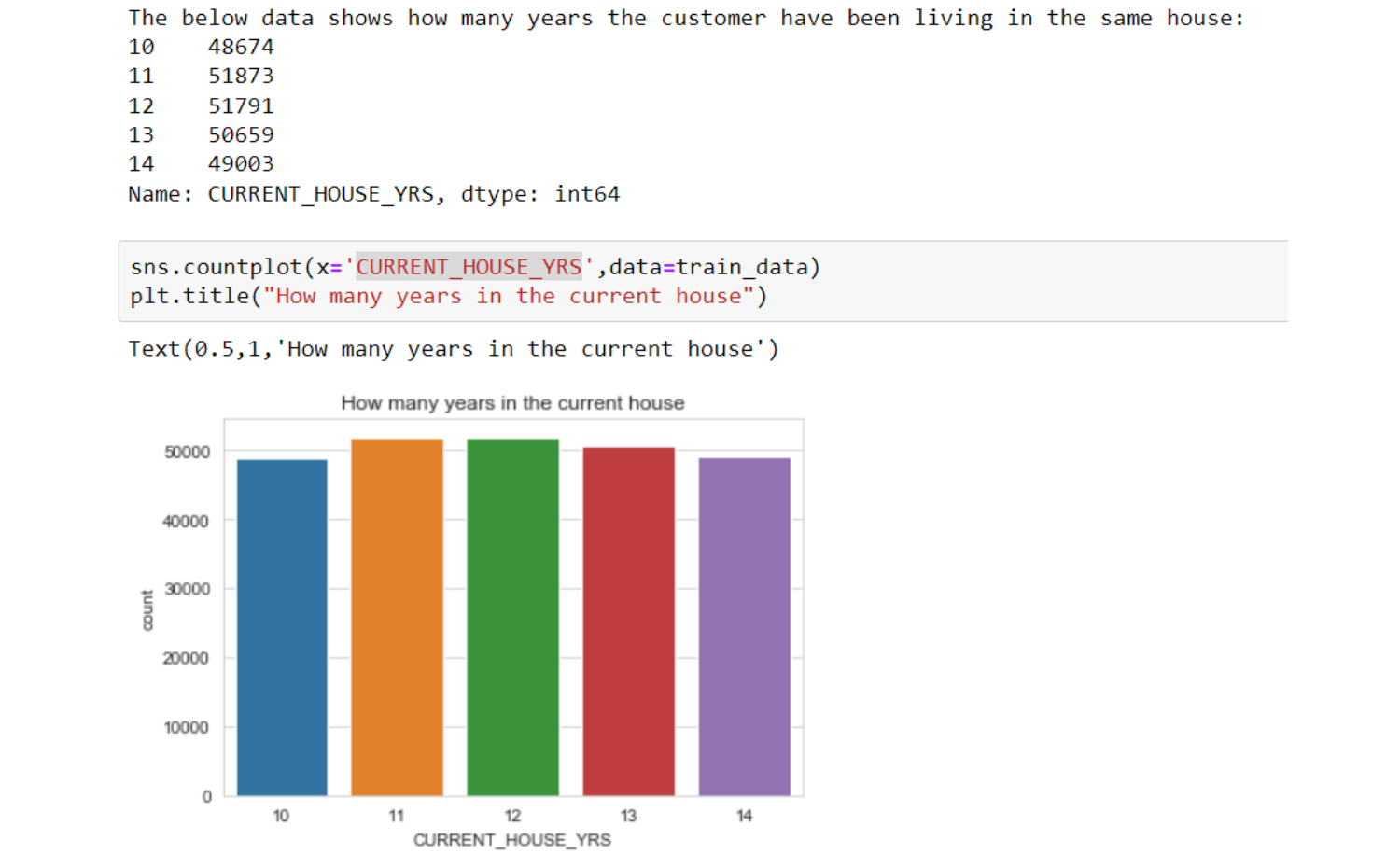
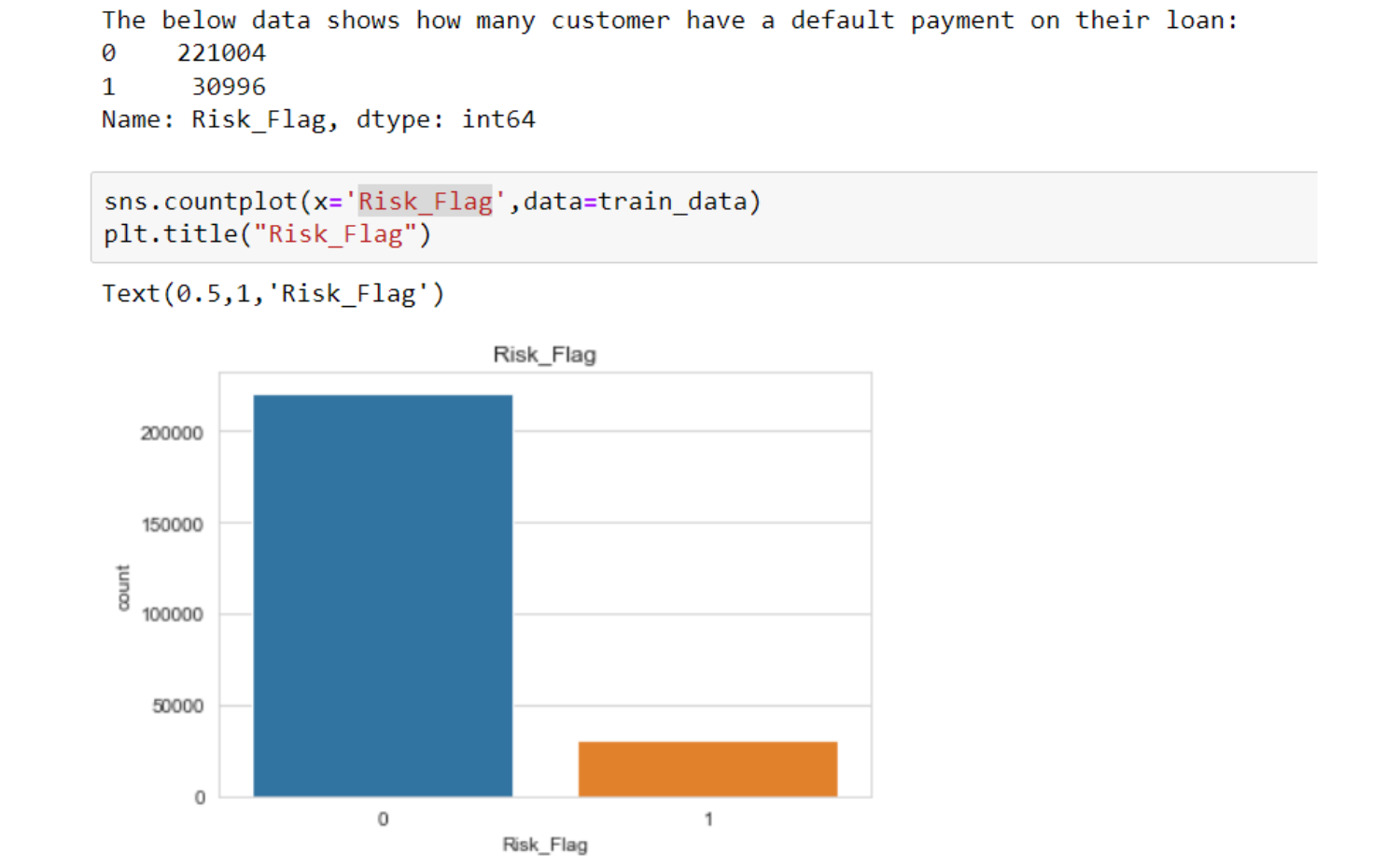
Observations
Most of the clients:- are single
- are renting
- don't own a car
- have been working at the same job for the past 3 to 8-9 years
- have been living in the same house for the last 10 to 14 years
- have not default on their loan
Machine learning
For this project I have used the Train_test_split machine learning from sklearn. You can find more info about sklearn, Train_test_split and more here.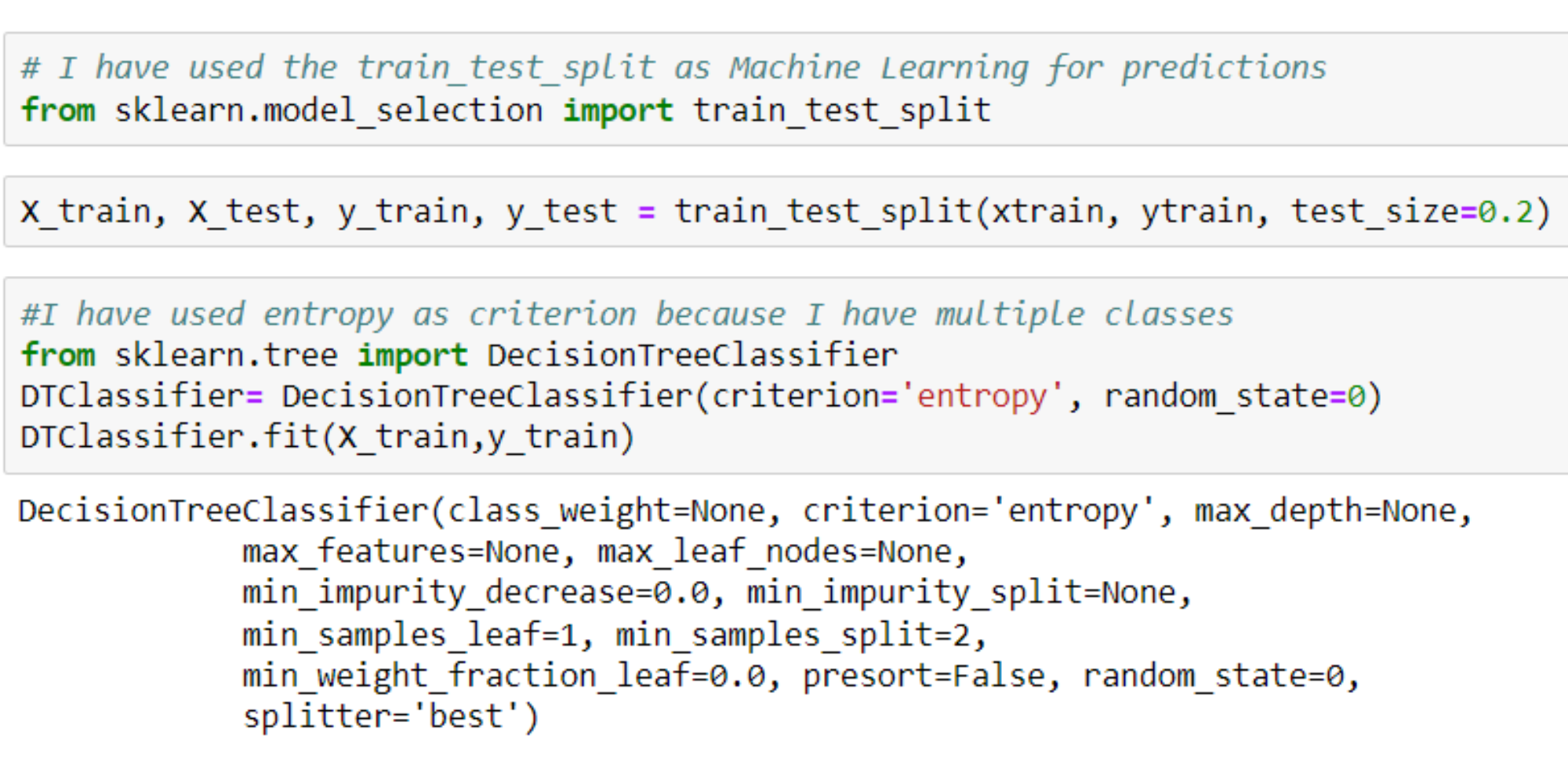
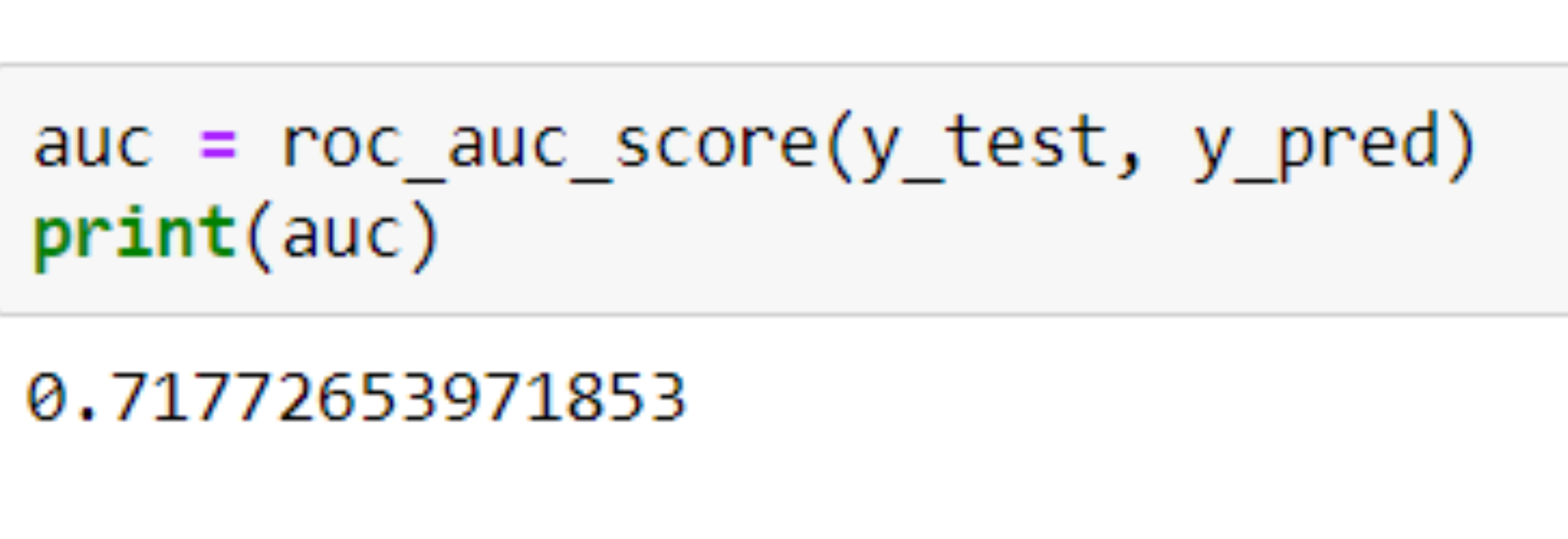